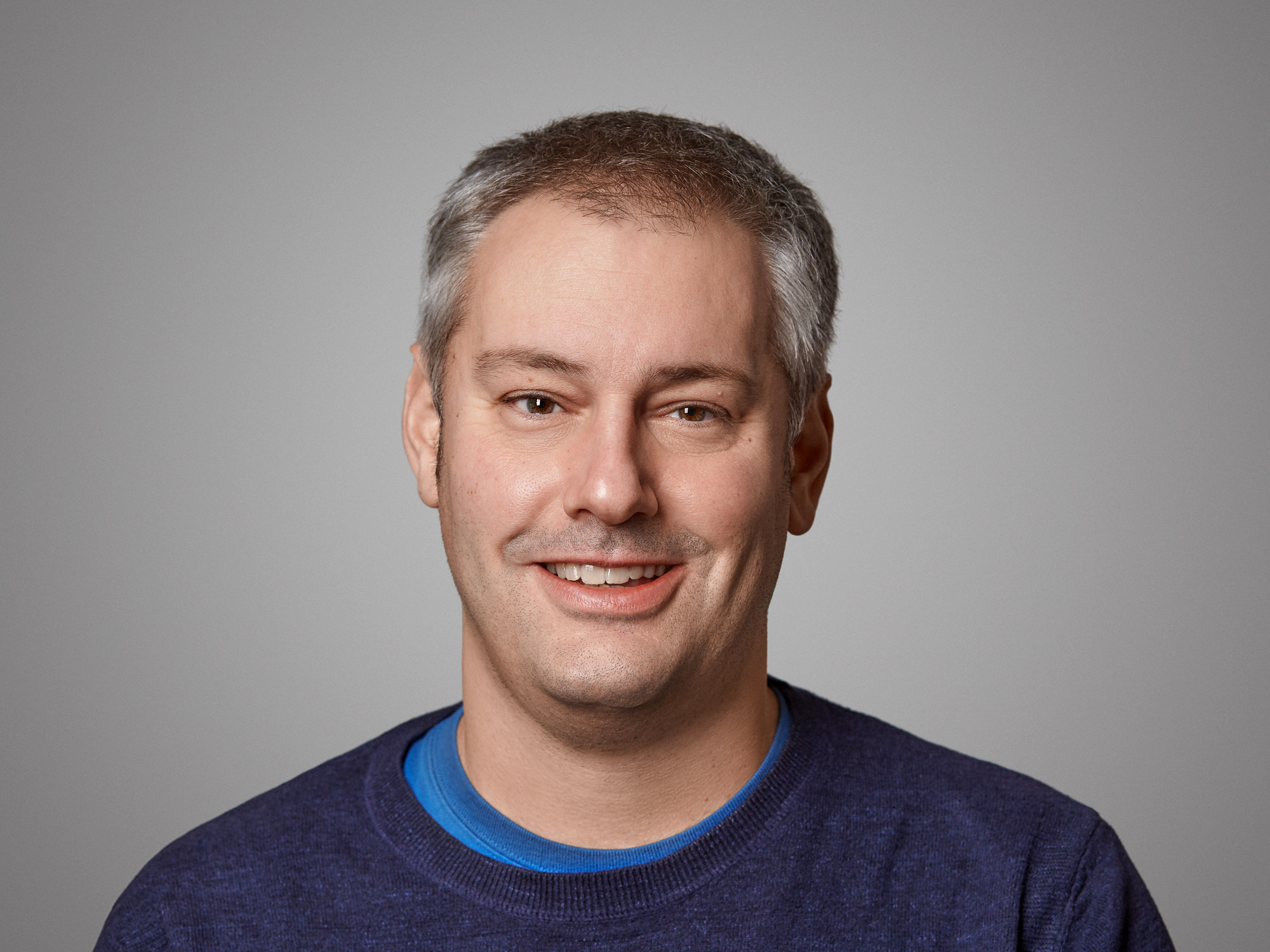
Sébastien Gambs
President,
CAIAC
Professor,
Université du Québec à Montréal
Privacy-preserving and Ethical Analysis of Big Data,
Canada Research Chair
In the last decade, the massive increase in the amount and the diversity of data collected about individuals, along with the advances in machine learning (ML) algorithms as well as the augmentation of computational capacity has enabled a “quantum leap” in the prediction accuracy in many domains.
These models can analyze complex information such as medical or graph data and have now become ubiquitous in many aspects of our society. However, their growing use in high-stake settings – such as college admission, recidivism prediction or credit scoring – also raise privacy and ethical issues (e.g., fairness and explainability). For instance, recent works have shown that even so-called black box models are vulnerable to privacy attacks that can infer information or even reconstruct part of the training set, which is often composed of personal information. In addition, discrimination arises because the training data is inherently biased for historical and societal reasons (e.g., toward an ethnic group or a vulnerable minority), in which case the ML model learns to reproduce this negative bias and may even reinforce it. Finally, their complex design makes it difficult to understand and explain their decision, which may lead to a lack of transparency and trust.
Canada has been at the forefront of the technical development of the Artificial Intelligence (AI) and ML, thanks to the development of the Pan-Canadian strategy on AI, the creation of the three AI hubs (i.e., Mila, Vector Institute and Amii) but also the research being conducted from coast to coast in academic institutions but also industries. A recent recognition about the impact of such research is the Nobel Prize in Physics being awarded to Geoffrey Hinton (University of Toronto) together with John Hopfield. However, the ethical issues mentioned previously but also more recent threats that have accompanied the rise of generative AI, such as the ease of creating deepfakes and fake news, have eroded the trust in AI and highlighted the need to ensure that its development is done in a manner that aligned with the society needs.
To tackle this challenge, Canada was already among the first countries to develop ethical guidelines with the Montréal Declaration for Responsible AI in 2018, followed in 2019 by the creation of the International Observatory on the Societal Impacts of AI and Digital Technologies (Obvia) in Québec. Since then, the research aiming at understanding and addressing the societal issues related to the development of AI has bloomed and many initiatives have emerged to raise the awareness about these issues. In particular, the Canadian AI conference (the national conference organized by the Canadian AI Association, CAIAC) has set in recent years a track dedicated specifically to Responsible AI research and the NSERC-funded program on the Responsible Development of AI aim at training graduate students on these topics.
Nonetheless, much work remains to be done to be sure to ensure that things really changed on the ground. In particular, while many companies have signed ethical charters for the responsible development of AI, these charters are not legally binding and thus it is not clear that the practice of companies have changed significantly. This could potentially lead to a form of ethics washing and calls for stronger approaches based on regulation, similarly for instance to the AI act in Europe. This regulation would help to ensure that these ethical issues are operationalized in a coherent manner while also fostering innovation by having a set of common rules for the development of responsible AI. In addition, it is now clear that AI systems are shaping how society evolves and constitutes a sociotechnological question, rather than only a technical one. Thus, there is a need to involve more research coming from the social sciences and humanities to shed light on how AI will impact the future of our society. Finally, there is also a growing need to include more training on the ethical aspects of AI to ensure that data engineers and ML are aware of these issues and know how to address them. Luckily, Canada has a vibrant ecosystem and the expertise to address these challenges.
To learn more, visit caiac.ca.