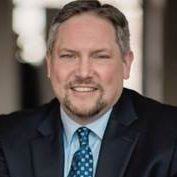
Matt Hoerig
President of Trustsec Inc., Vanguard Cloud Services, and the Cloud Security Alliance Canada
Last year, Matthew Hoerig from CSA Canada provided a high-level look at a broad-based approach to AI security. This discussion touched on ethics, integrity of the data, version control, and data validation. This year, Matt has decided to go a step more in-depth and look at the AI generative model with a deeper lens on security.
Current, widely available AI tools such as CoPilot, Chat GPT, Gemini and Meta AI all generally consist of a similar architecture; Encoders, GAN’s (Generative Adversarial Network’s; generators /discriminator’s), Transformers (query translation), and LLM’s (Large Language Models) for content generation.
A key security concept here to keep in mind is the quality or the integrity of the data inputs. The larger the environment being ‘scraped’ for content and query response, the more chance of inaccuracy in the output. Or another way to look at it, the smaller the data source the more accurate the query response will be.
In the case of Government, there are scenarios where generative AI tools may be used to support applications either internally or serving the public. Those AI tools may be querying, transforming, and generating content locally within government networks or the whole of the internet depending on the nature of the application. All the usual security considerations here should be top of mind, including access control, data sensitivity (unclassified vs. elevated categorization), data poisoning, and inversion attacks.
Data integrity is considered one of the most significant risks when considering generative AI output. Bias is a key threat to the data enrichment process, and of course the desire to leverage the output, whatever the usage scenario may be. The Canadian Government (Treasury Board Secretariat) has developed an assessment process for automated decision making which may impact the algorithmic design and use of AI systems in government. This is a required assessment process for the intro and use of any AI system for GC departments and agencies.
At the end of the day, AI is simply another tool or application with inputs and outputs and data security best practices; access control (RBAC & least-privilege models), data-loss prevention, network security (NIST/ITSG zoning models and micro-segmentation where software-defined networking [SDN] may be in place), and expansive audit logging and monitoring are all effective security and risk mitigation elements which should be in place prior to the use of AI systems. These security areas of focus become easier to provision and consume when the AI system resides in the cloud. As is the case based on current trends. Increased use of AI as an organizational tool is inevitable, whether it be RPA (Robotics Process Automation) or leveraging any of the generative AI tools mentioned at the beginning of the article. As long as appropriate security controls are in place in support of those systems the ubiquitousness of AI will continue to grow as an effective business tool across government organizations.
Learn more at csacanadachapter.ca.